Robotics, Automation and Machine Vision
Our Research
The team develops advanced robotic sensing technologies to enhance autonomy in crop production, biosecurity and animal welfare on-farm, with aims for on-farm adoption in the commercial farm conditions of today. The team also provides leadership on the integration of sensing systems with agricultural robotics into the future.
Primarily the advanced sensing systems involve machine vision technology, which also incorporates supporting mechanical structures for on-farm installation and deployment, electronic interfacing with controllable outputs like spray nozzles, drafting gates or remote notification in real-time and fusion of multiple digital data sources and decision support systems.
Our People
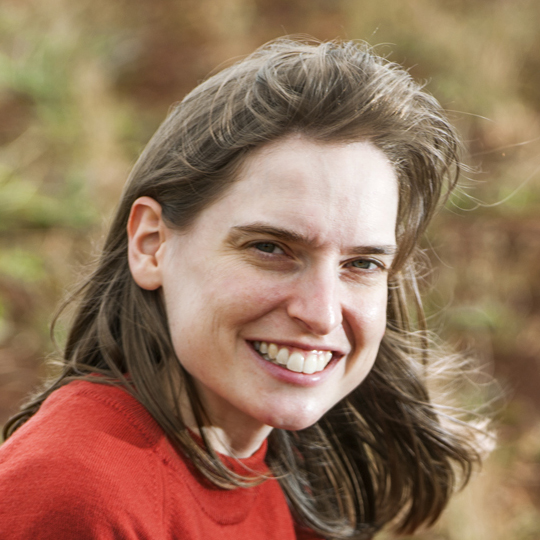
Dr Cheryl McCarthy
Senior research fellow
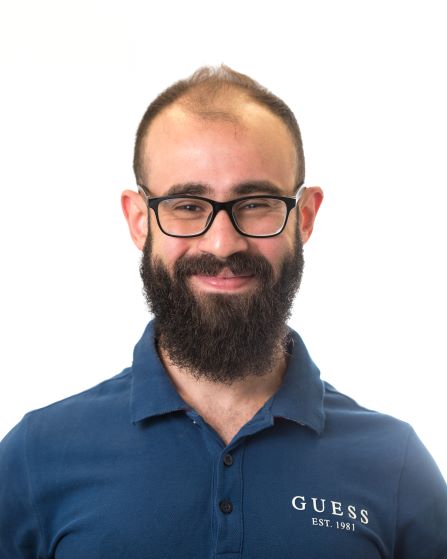
Basem Aly
PHD student
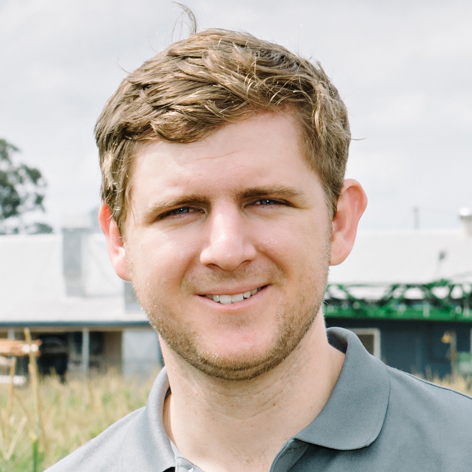
Dr Derek Long
Lecturer / Researcher
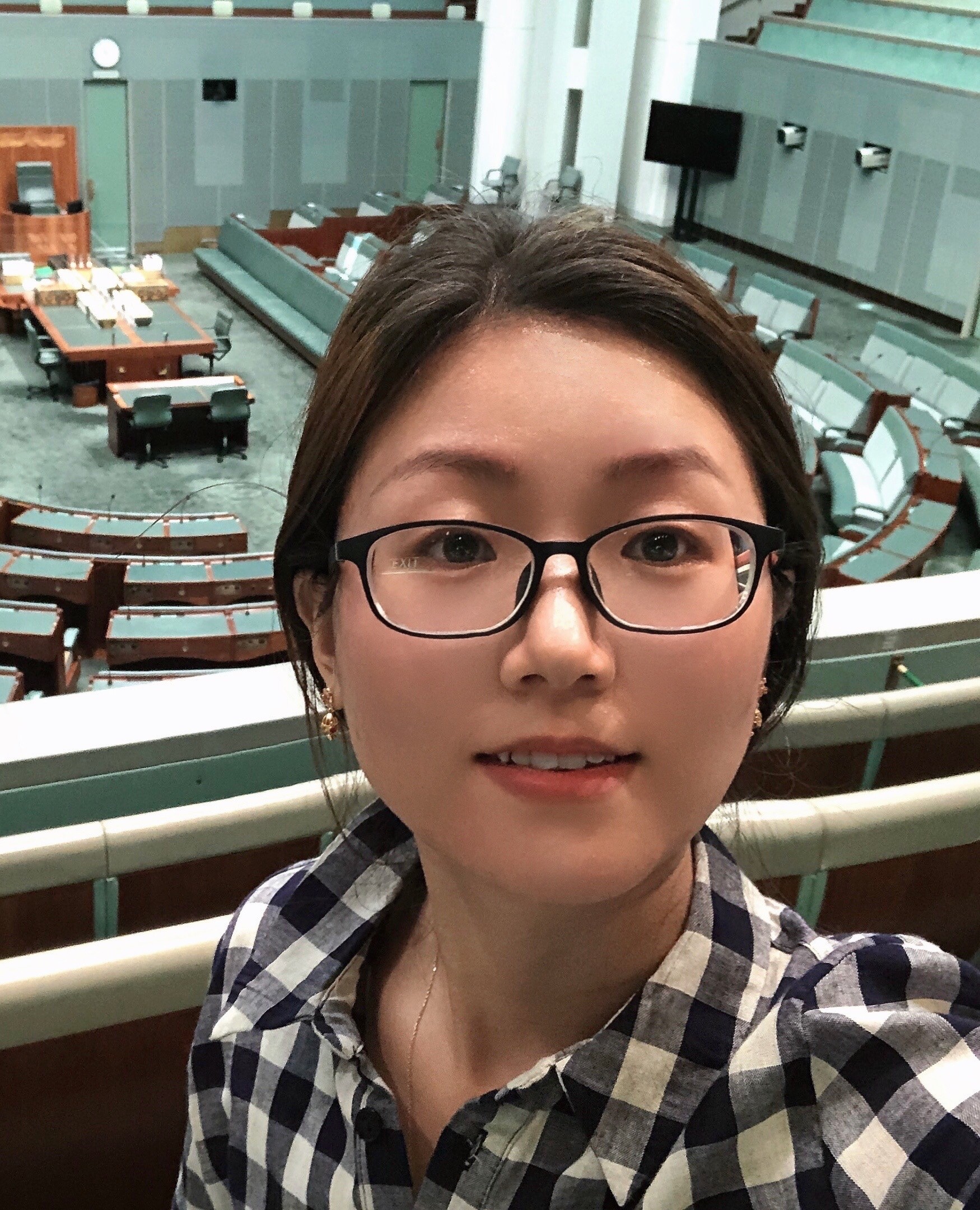
Yiyi Xiong
PHD Student
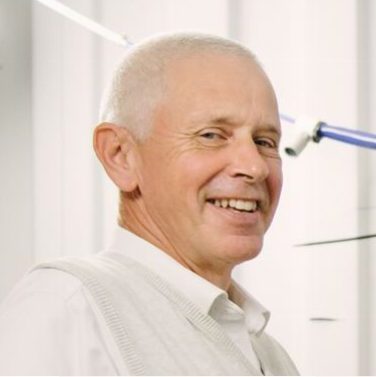
Prof Peter Brett
Professor (Agricultural Technologies and Robotics)
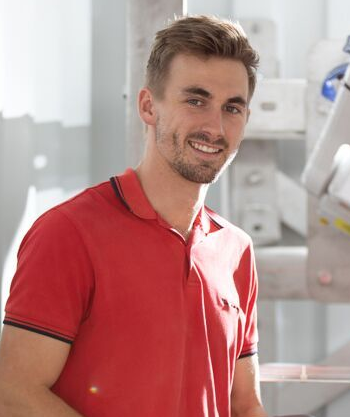
Fraser Border
phd student
Key Projects
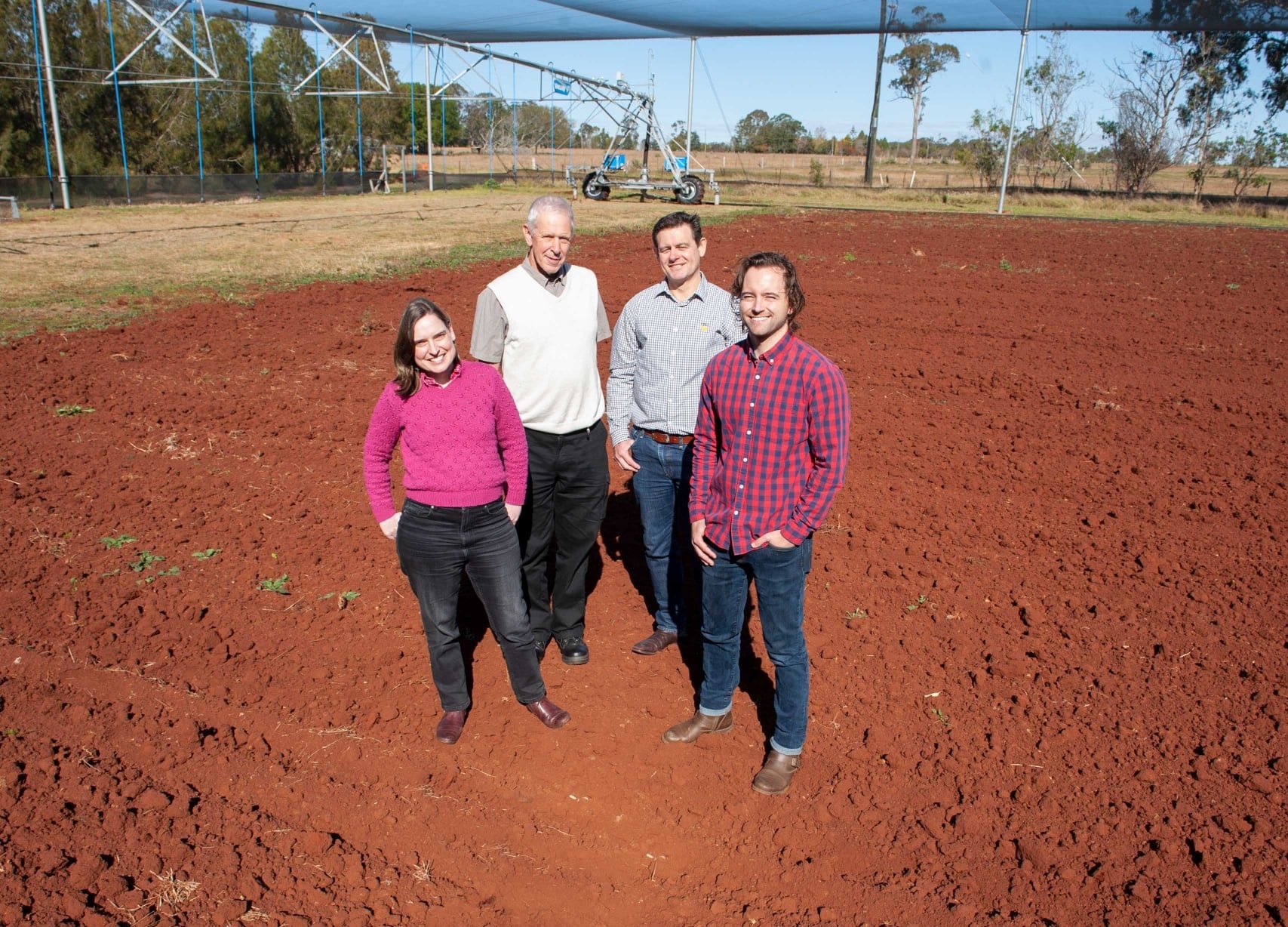
Moon to Mars initiative
Our research project will develop launch-ready software for automated monitoring of plant stress signals, so fresh vegetables and leafy greens can be grown at the International Space Station (ISS) with increased food safety and providing new food options for astronauts. The launch-ready software will use machine vision sensors for plant stress and will be developed during laboratory experiments at USQ in collaboration with NASA and partner organisations. The software is intended to be deployed on space flights during the mission phase, enabling growing of healthy food plants with minimal to no crew interaction. The project will produce a larger ground-based laboratory in parallel with the launch-ready software, enabling the investigation of Space Agriculture Systems for deployment to planetary surfaces in the future.
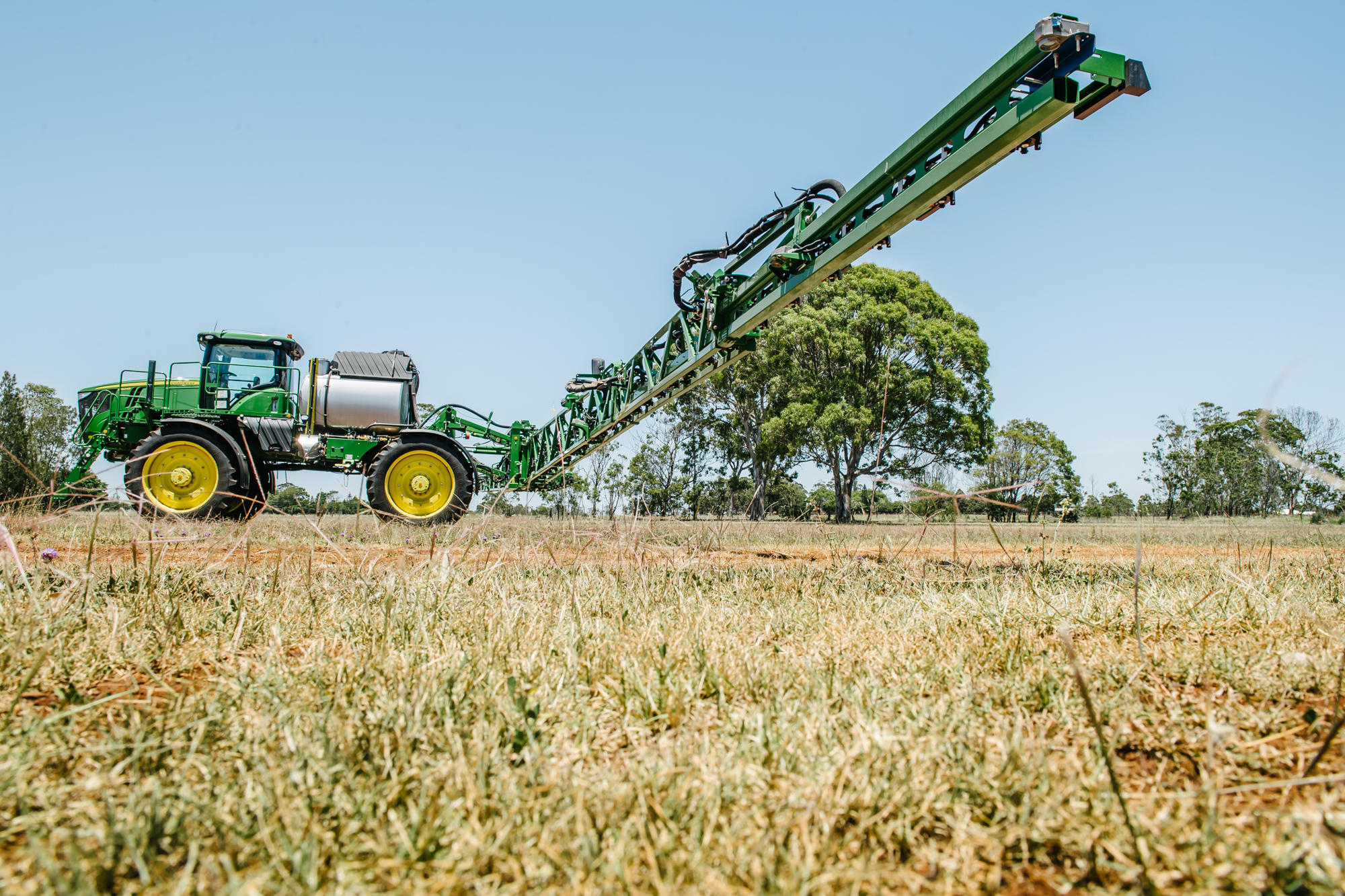
Precision weed map generation for weed spot-spraying in cotton
Development of a weed spot spraying field prototype / proof-of-concept. The prototype will be evaluated as a low-cost and high-accuracy alternative to existing commercial technologies for spot spraying weeds in a fallow situation. Compared to current commercial technologies, this system will offer superior weed detection by separating grass weeds from broadleaf weeds. Broadleaf weed detection will be further refined to distinguish cotton plants, providing additional flexibility in fallow situations while also paving the way for weed detection within cotton crops. The technology will enable growers to implement weed management strategies that reduce herbicide usage.
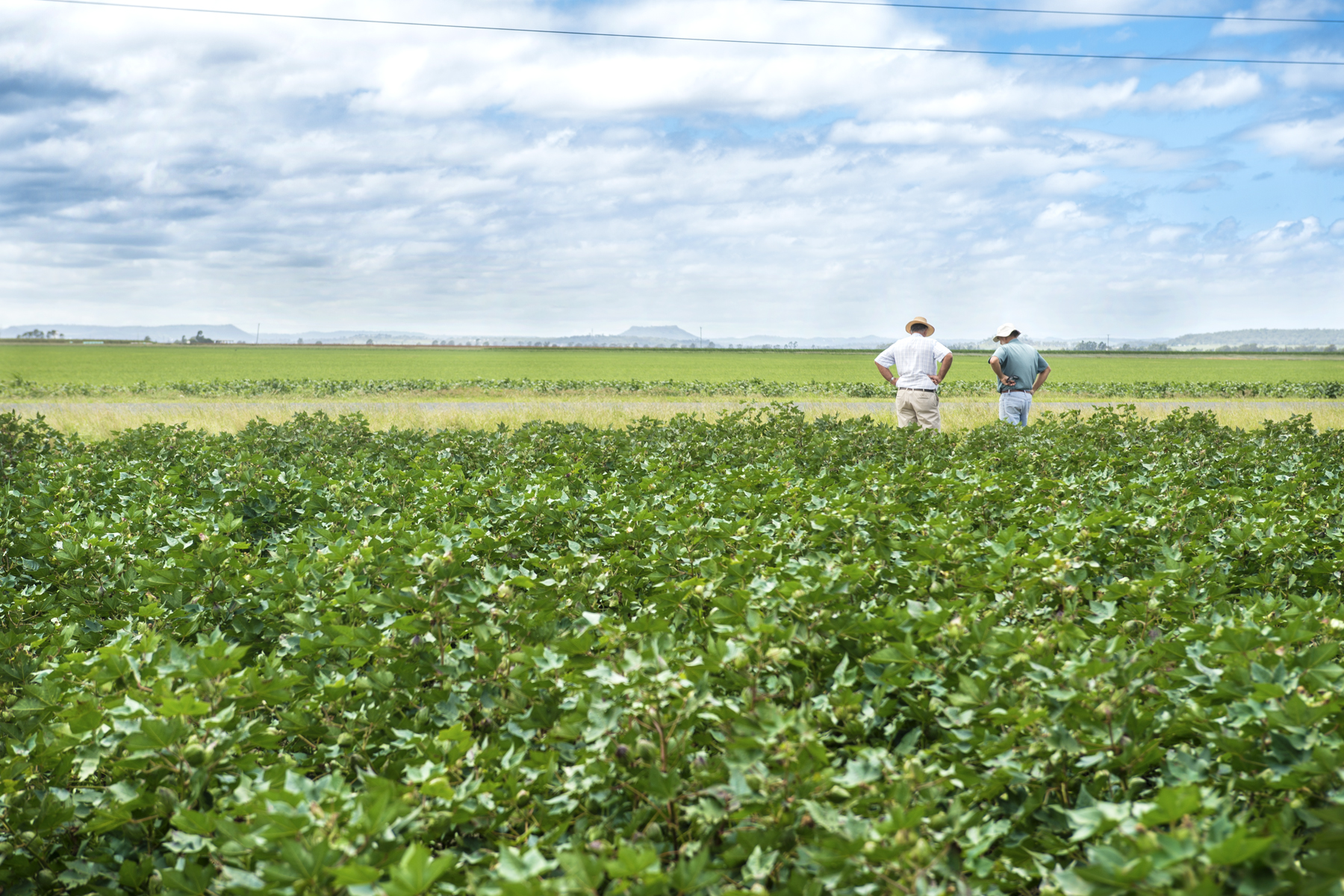
Precision management for improved cotton quality
The project will identify opportunities (and the associated sensor and data collection requirements) to improve both the overall quality and consistency of Australian cotton through an improved ability to assess (predict and model) and manage crop and cotton quality development. The project will utilise previously developed expertise in managing specific parts of the production pipeline that are known to influence fibre quality. Emphasis will be placed on establishing the fibre quality of crops at defoliation and then managing harvesting and ginning to maximise cotton fibre quality.
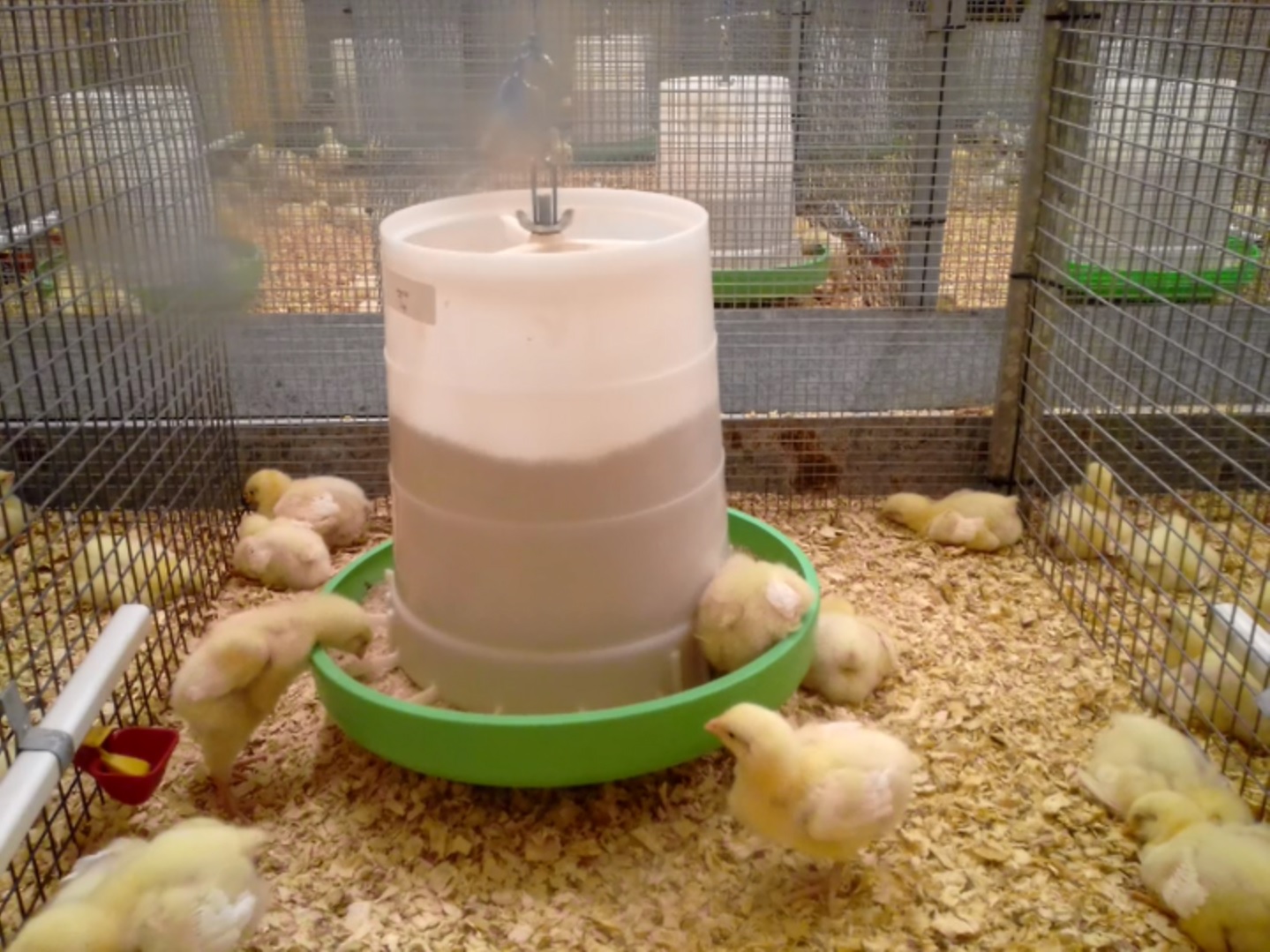
Novel detection of chicken welfare using machine vision
This project will develop a proof-of-concept, low-cost sensing and notification system based on image analysis algorithms for detecting chicken behaviour for animal welfare. A machine vision system on an autonomous platform has potential to automate detection of dead chickens, and to record the cage location for subsequent removal of the dead chicken by a human handler. A preliminary study was conducted in 2018 which demonstrated discrimination of dead chickens using a machine vision system and recommendations for the further work that is now progressing.
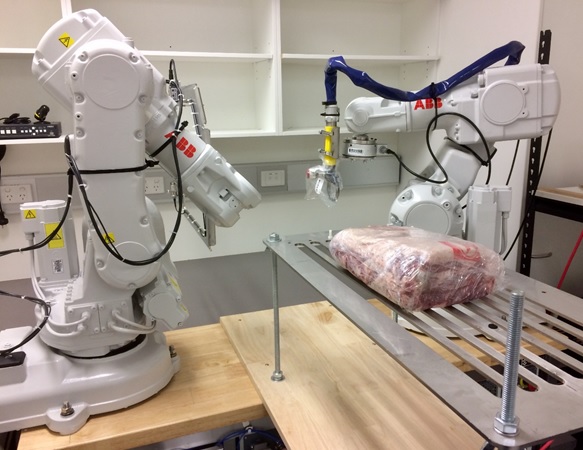
Motion and Time - Average timing of stages in the deboning of beef carcasses
Project with Oakey Beef Exports (OBEX) to determine the average and standard deviation of the time taken to achieve specific cuts of meat sections from a beef carcass by skilled butchers working on a production line. The outcomes of this project will allow OBEX to explore re-evaluation of agreed time periods in view of the many improvements made on the production line since the 1960’s and to therefore maintain a sustainable and competitive position in the meat processing market.
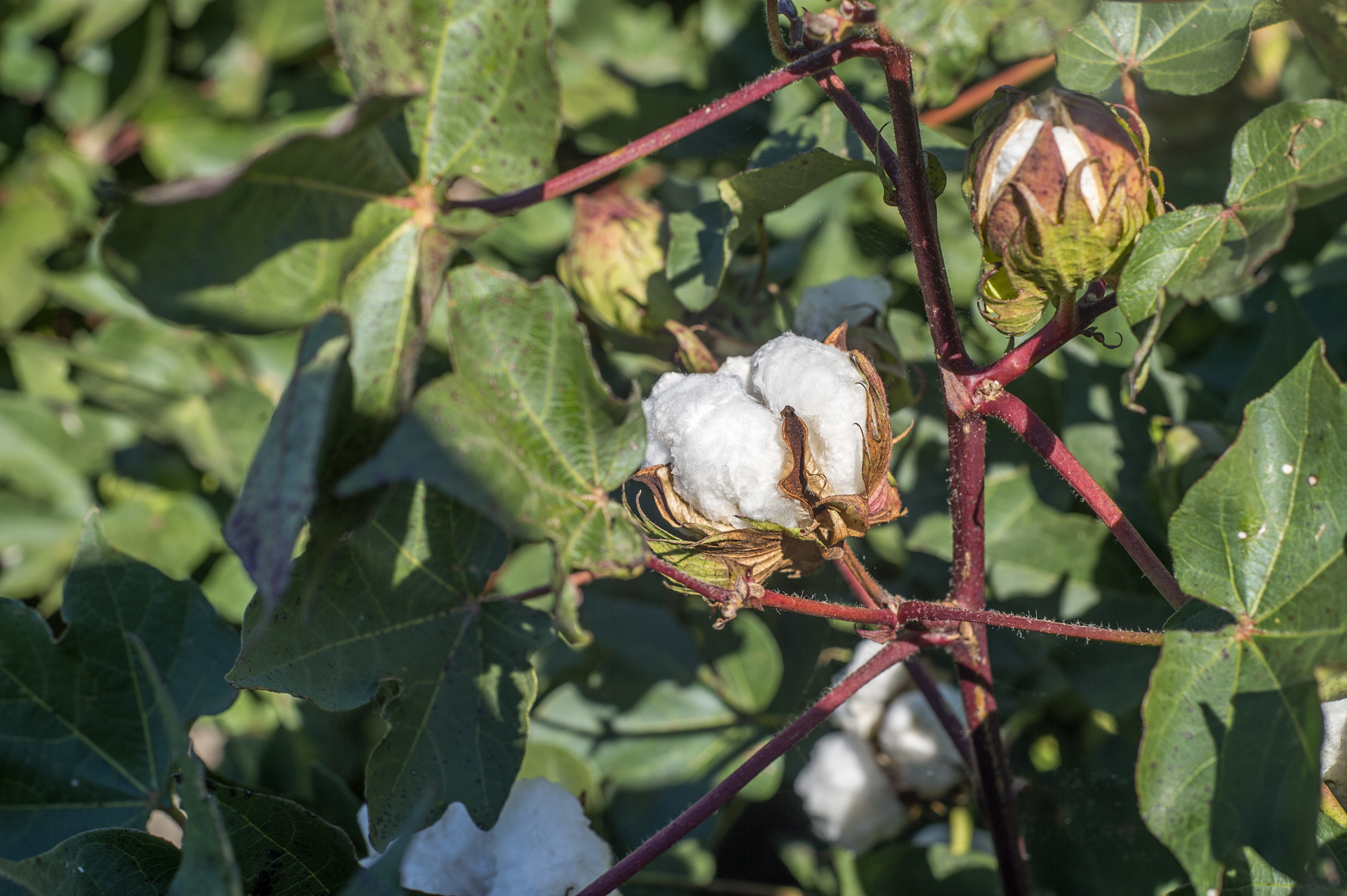
Improved pest management in cotton
The project will seek to explore the potential for sensing technologies and machine learning to be used for cotton pest detection and quantification and how such information might be used to inform pest management decision making. Benefits to the industry and community include rapid and accurate collection of diagnostic data that can be used for better decision making; optimising labour use; and optimising deployment of control practices that may result in less pesticide usage within the environment and reduced selection pressure for resistance.
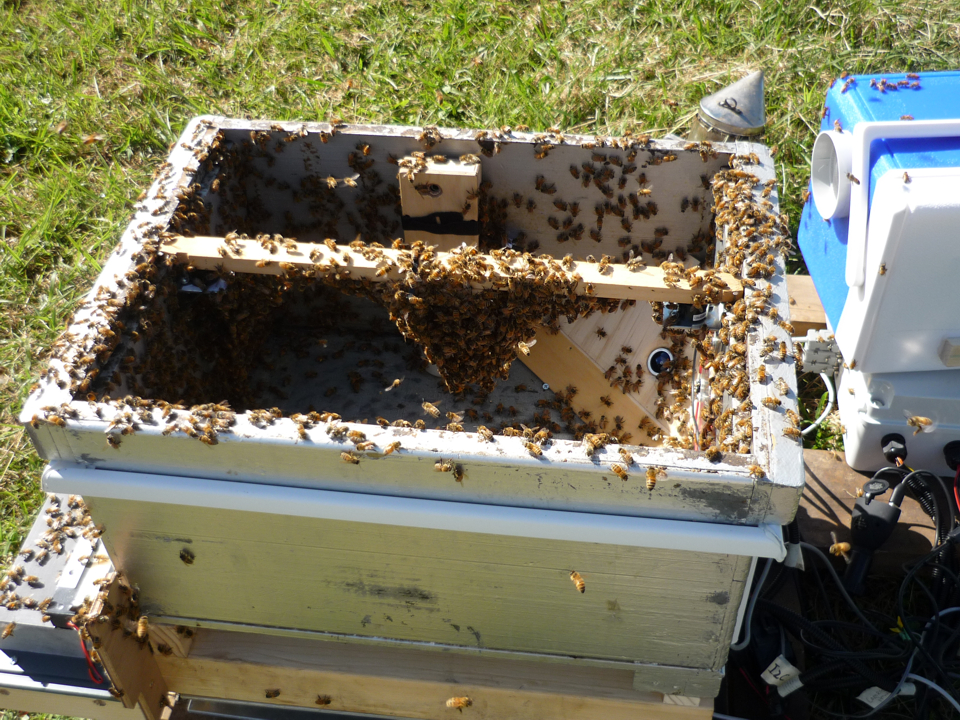
Remote catchbox deployment
An extension to work previously completed under the bee pest surveillance program. This project focuses on the refinement, development and deployment of improved Remote Catchboxes (RCBs) and ongoing service of the RCBs as part of the overarching National Bee Pest Surveillance Program. Project will begin with a trial of the refined RCB in Queensland monitored by QDAF & USQ, and if successful will then require 40 improved RCB’s to be deployed nationally and monitored. This will enable long‐term efficiency in data collection and sensitivity to capture bee swarms with remote catchboxes and expand the surveillance coverage in areas that are either geographically remote or with restricted access of high risk concern.
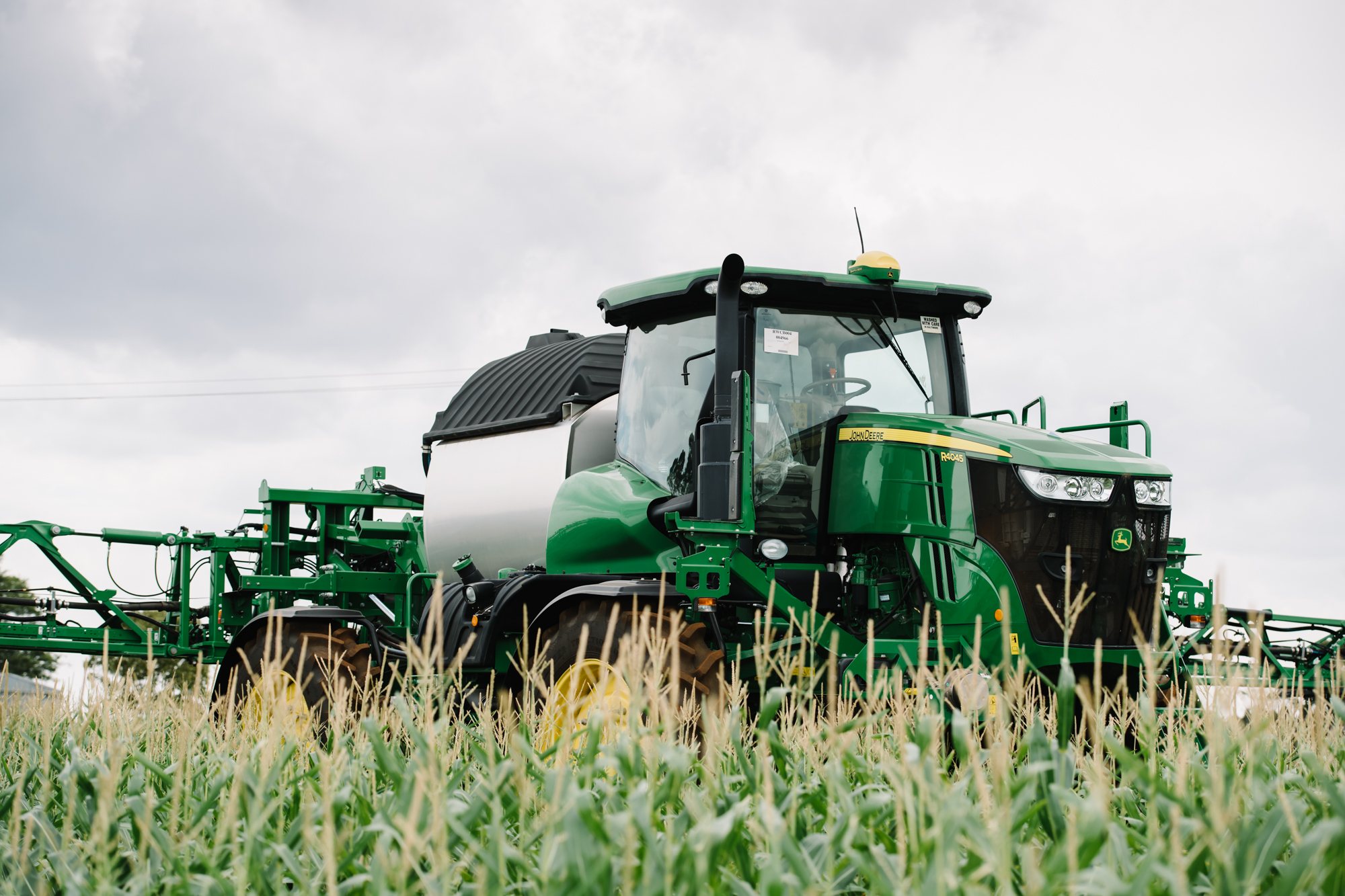
Future Farm Phase 2: Improving farmer confidence in targeted nitrogen management through automated sensing and decision support
The Future Farm Program aims to re-examine and improve the way in which soil and crop sensors are used to inform decisions about input management and to provide a way of automating the process from data acquisition, through analysis, to the formulation and implementation of decision options. It is expected that by 2025, Australian grain growers will be generating a minimum 15% increase in return on investment from nitrogen fertiliser applications in winter cereals and by 2023, the generation of the knowledge, datasets and IP required to develop automated sensing and management applications for various end-use applications in farming systems and tactical agronomy.
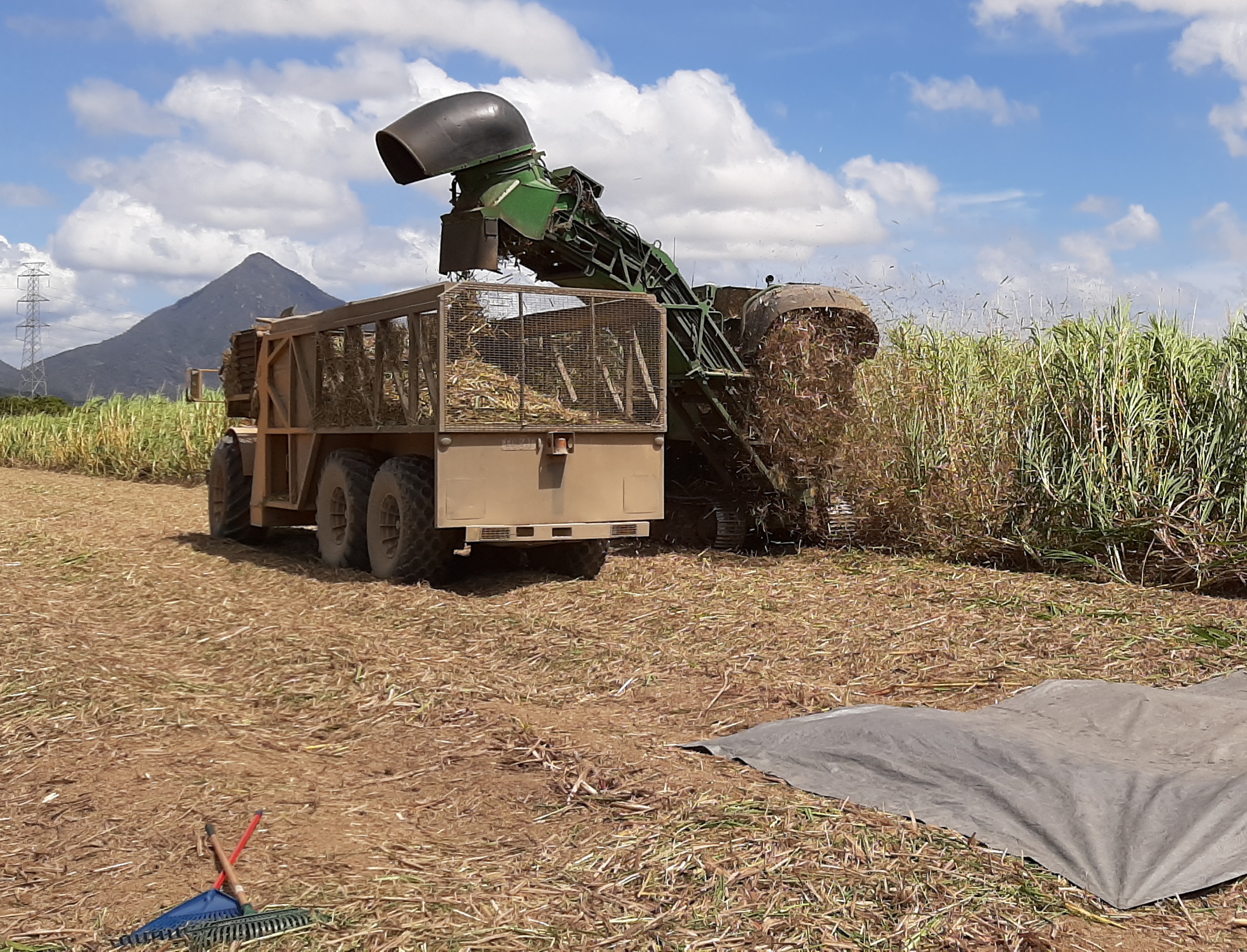
Sugar Research Australia: Harvester losses
Sugarcane losses from harvesting is estimated at 10% to 40%. Factors such as the speed of the harvester and the speed of the extractor fan affect how much extraneous matter is retained, and the quantity of billets and sugar cane juice lost during harvesting. The project will develop a novel proof-of-concept Machine Vision System for real-time estimation of cane losses during harvest (i.e. imaging to detect billet particles and juice that falls onto trash blanket). Hence, the harvester operator can potentially adjust harvester operational parameters and amount of loss in response to real-time conditions.
Posters and Flyers
Research Partners
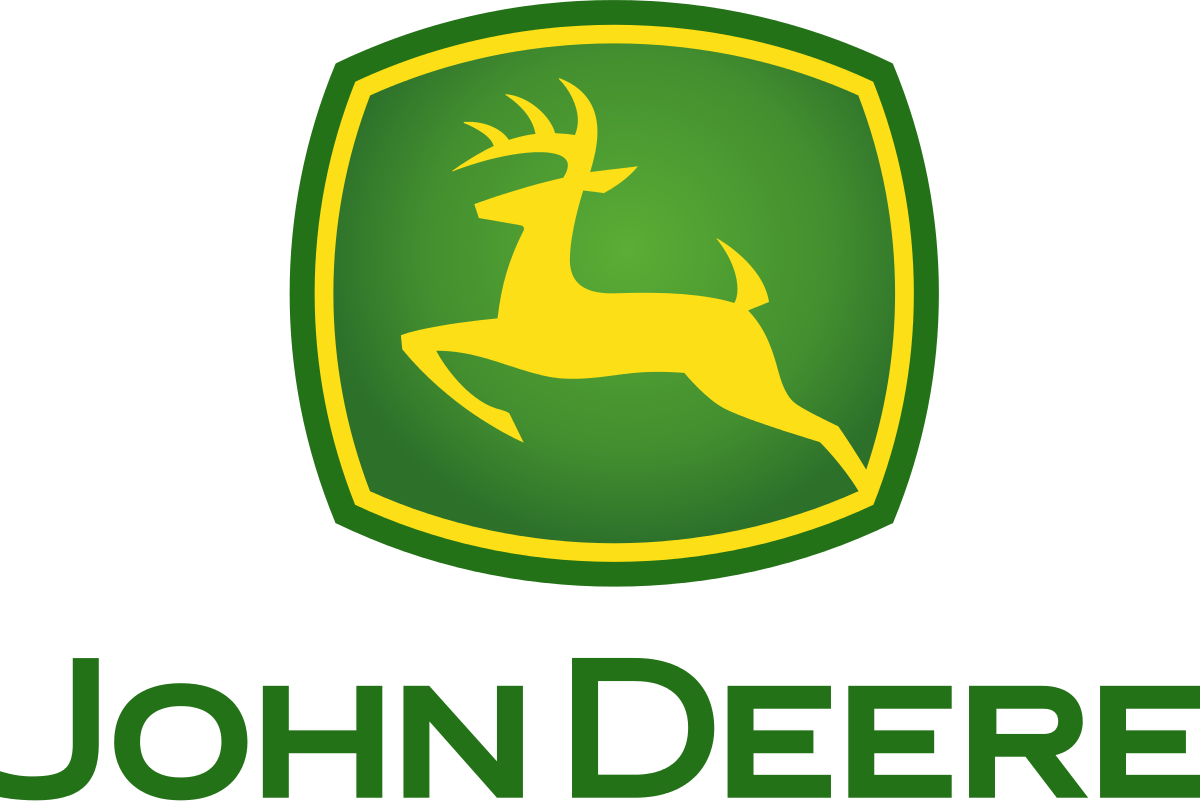
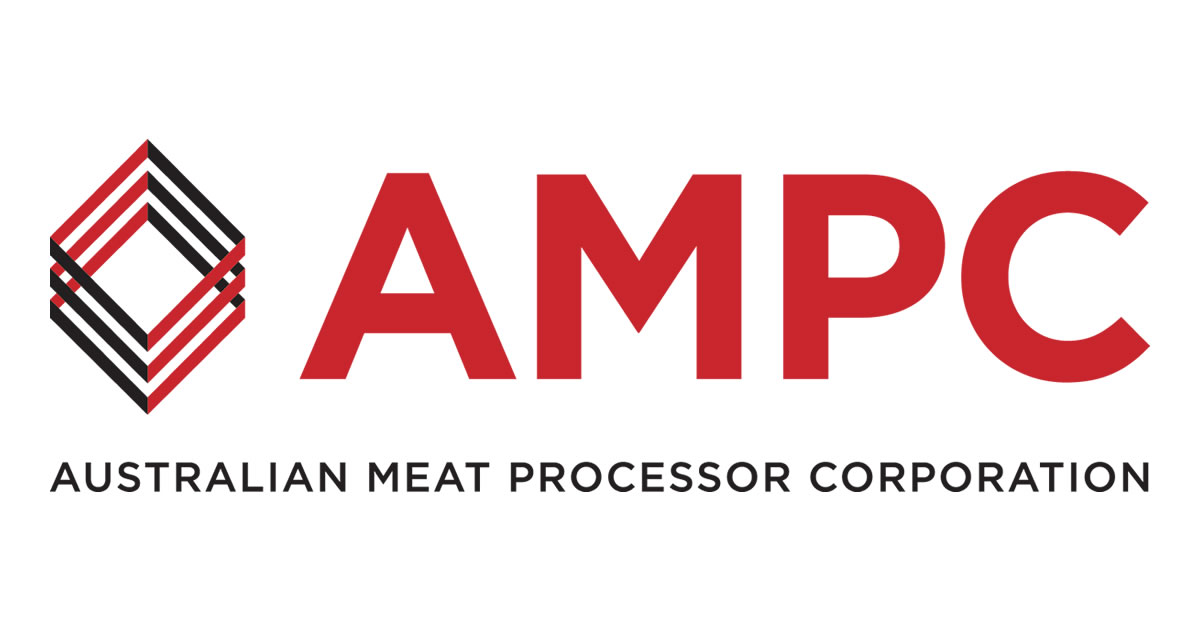


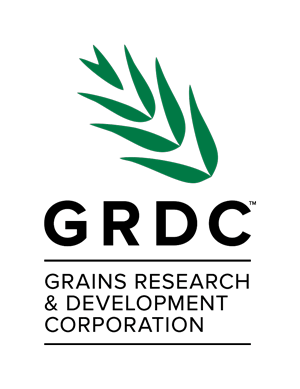
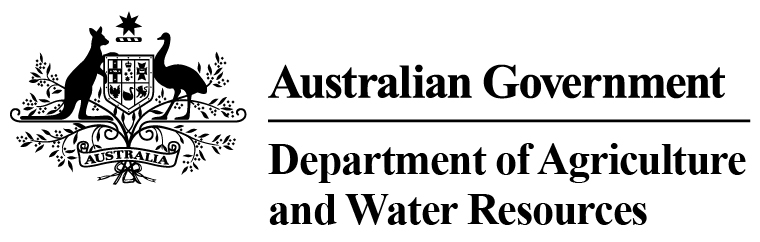
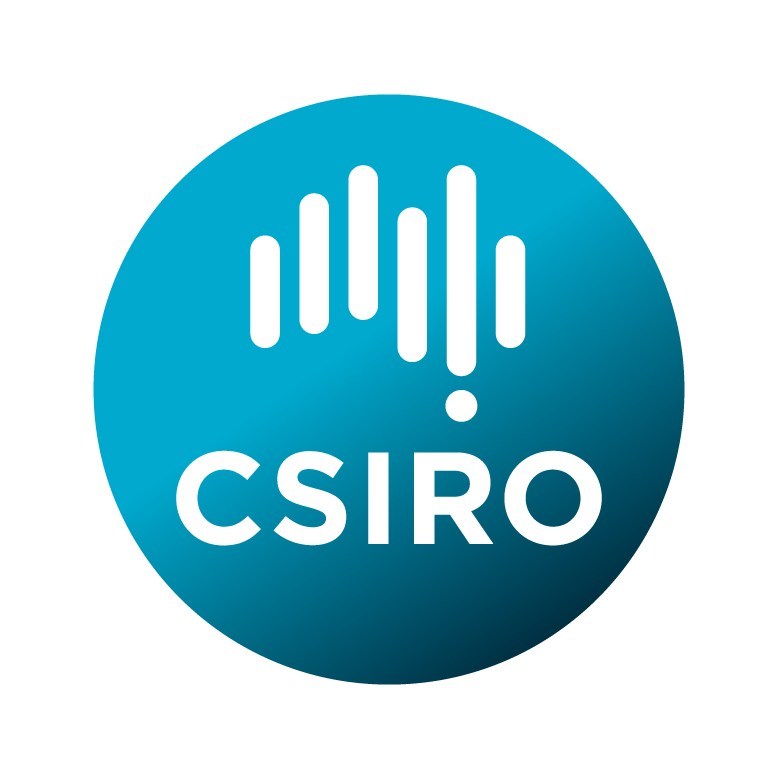
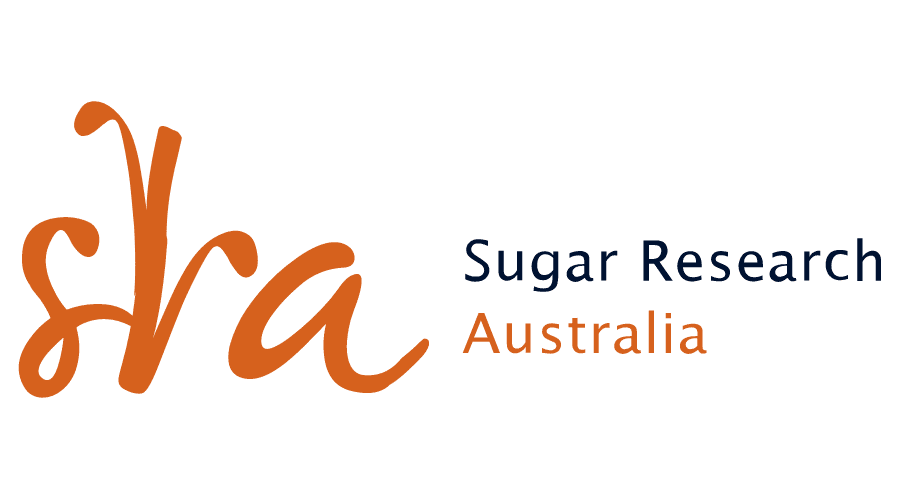
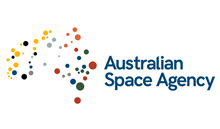